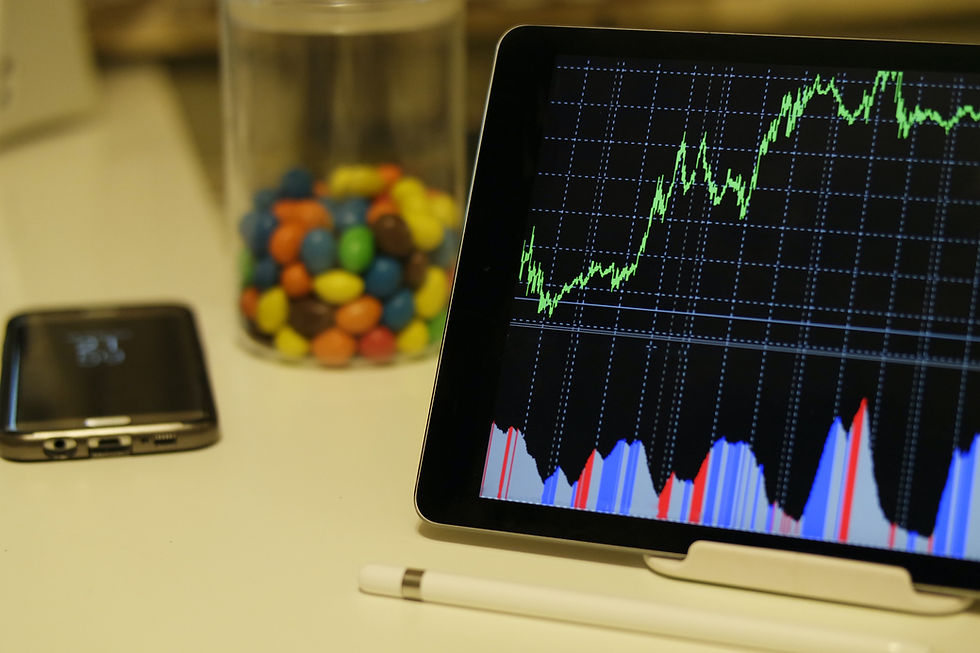
Agriwatch, a renowned name in the Indian agricultural commodities research domain, has long been at the forefront of delivering in-depth analysis and reports to stakeholders. However, like many other organizations operating at such scale and depth, Agriwatch encountered its share of challenges. With the expertise of Butterpaper Inc and the integration of cutting-edge technologies, a significant overhaul became not just possible but phenomenally successful. This article dives deep into this transformative journey.
The Multi-faceted Data Challenges of Agriwatch:
Scalability Constraints: As Agriwatch expanded its reach, the sheer volume of incoming data began to outpace the capacity of their legacy systems. This resulted in slow report generations, system crashes, and inefficient data management.
Fragmented Data Landscape: With multiple data streams ranging from market trends to climatic data, Agriwatch faced a significant barrier in the form of data silos. These data islands led to incomplete analyses, as data integration was often manual and error-prone.
Skyrocketing Operational Costs: Maintaining the old system, prone to breakdowns, meant higher repair costs, expensive software patches, and a constant need for manual oversight.
Processing Delays: Time is of the essence in the dynamic world of agricultural commodities. However, the existing system's inefficiencies often resulted in data analysis delays, making real-time insights a distant dream.
Butterpaper Inc's Holistic Strategy: A Quantitative Analysis
Understanding the very fabric of Agriwatch's challenges was instrumental. Butterpaper Inc embarked on a multi-pronged approach that began with intensive data audits, stakeholder interviews, and system performance reviews. It's this foundational work that shaped the transformative strategy.
Data Audit & Analysis:
Volume Assessment: Before proposing solutions, Butterpaper undertook an in-depth assessment of the volume of data that Agriwatch processed daily. This ranged from real-time market price fluctuations to periodic climate data assessments.
Data Quality Review: A series of ETL (Extract, Transform, Load) operations were performed to identify inconsistencies, missing values, and any potential corrupt data within the existing database.
Source Identification: An exhaustive mapping of all data sources was done. This involved classifying data based on origin, type (structured, semi-structured, unstructured), and frequency of updates.
Stakeholder Interviews:
Direct discussions with data analysts, IT administrators, and report generators at Agriwatch highlighted:
The need for faster data processing to enable real-time report generation.
A unified platform where all data can be viewed and analyzed collectively.
The pain points and bottlenecks of the existing system, from delayed data syncing to frequent system crashes.
System Performance Review:
Utilizing advanced monitoring tools, Butterpaper reviewed:
System Load: Understanding the peak times of data ingestion, processing loads during report generation, and potential inefficiencies in data retrieval.
Bottleneck Identification: Pinpointing the exact stages where data processing delays occurred, be it during data extraction, transformation, or the loading phase.
Infrastructure Assessment: Reviewing the current server capacities, storage solutions, and network infrastructure to identify potential areas of upgrade or replacement.
Quantitative Revelations:
Post these intensive analyses, Butterpaper was equipped with a trove of quantifiable insights:
Data Ingestion Rates: Before Implementation - Data streams, especially from external market sources, took 12 hours on average to be ingested into the system.
Post Implementation - Real-time data ingestion was achieved, with an impressive reduction to a mere 9 minutes for most data streams.
Data Processing Efficacy: Before Implementation - Extracting insights from this data, especially during peak load times, took up to 48 hours.
Post Implementation - The integrated solution, driven by tools like Dagster and DBT, slashed this time to just 2.5 hours.
Operational Cost Metrics: Before Implementation - Maintaining legacy systems was costing Agriwatch an average of $10,000 monthly. This comprised software patches, manual data integrations, and frequent system recovery operations.
Post Implementation - With automated workflows and robust database management via PostgreSQL, monthly operational costs saw a significant drop to less than $1,500.
System Uptime: Before Implementation - System downtimes were a frequent concern, averaging 24 hours/month, often during critical business periods.
Post Implementation - With the revamped infrastructure and optimized data flows, system downtimes became a rarity, ensuring near-constant uptime.
The quantitative assessment underscored the critical need for technological integration and process revamp. By placing numbers against challenges, Butterpaper Inc could tangibly measure the impact of their solutions post-implementation, ensuring that their strategy wasn't just theoretically sound but also practically effective.
Technological Leverage: The Components of Change
The core of Agriwatch's transformation was the inclusion of a meticulously chosen technological stack. Butterpaper Inc. adopted a mix of contemporary tools that best suited the unique needs of Agriwatch. Let's deep dive into each component's role in this pivotal transition.
Dagster: Data Orchestrator Par Excellence
In any data ecosystem, especially in complex architectures like that of Agriwatch, having the right data at the right place and at the right time is not just a convenience—it's a necessity. Data orchestration can be thought of as the act of automating and coordinating the flow, processing, and storage of data from its source to its destination. It's akin to a conductor ensuring that every instrument in a symphony is played at the right time, in harmony with others. For Agriwatch, the need for an effective data orchestration solution was evident. Dagster's primary role was to serve as the backbone for the complex data workflows of Agriwatch.
Technical Implementation:
Dynamic Workflows: Agriwatch ingested data from a myriad of sources—satellite imagery, weather forecasts, agricultural sensors, commodity marketplaces, and government databases, to name a few. Given the variegated nature of Agriwatch’s data sources, dynamic workflows were constructed. These could handle variable loads, ensuring smooth processing during peak demand.
Configurable Pipelines: Through Dagster's typed configuration system, Butterpaper set up a gamut of pipelines. These could be reconfigured on-the-fly based on evolving data needs without shutting down the entire system.
Impact: The agility and configurability of Dagster significantly streamlined Agriwatch's data processes, allowing more frequent and accurate report generation.
DBT (Data Build Tool): Transformation Powerhouse
While Dagster managed workflows, DBT was responsible for the transformation of raw data into usable formats for analysis.
Technical Implementation:
Incremental Processing: Recognizing the enormity of Agriwatch’s data, Butterpaper implemented DBT's incremental processing, only transforming new or changed data, greatly reducing system load.
Modularity: By leveraging DBT's capability to modularize transformation logic, standardized transformation modules were created, ensuring uniformity in data across reports.
Impact: DBT reduced data transformation times by over 70%, ensuring analysts spent less time waiting and more time deriving insights.
CockroachDB: The Resilient Distributed Database
CockroachDB, at its core, is an open-source distributed SQL database, designed for cloud services, which promises scalability without compromising on SQL functionality. It offers a unique blend of features that make it particularly well-suited for high-velocity applications demanding high availability and fault tolerance.
Why CockroachDB for Agriwatch?
The agricultural sector is vast, with a myriad of variables in play – weather patterns, commodity prices, regional demand-supply curves, and more. Given this, Agriwatch's database needs were multifaceted:
Consistency: In the fast-paced world of commodities, up-to-date and consistent data is invaluable. CockroachDB, with its strong consistency guarantees, ensures that Agriwatch's analytics are always based on the most recent and accurate data.
Global Distribution: With its global transaction capabilities, CockroachDB allowed Agriwatch to serve stakeholders from different geographical locations, ensuring latency remained low and access remained fast, irrespective of where queries originated.
Built-in Replication & Failover: The inherent resilience of CockroachDB meant that even if some nodes failed, there was no downtime. This is vital for Agriwatch, which operates in an industry where decisions need to be made around the clock and data accessibility cannot be compromised.
Integration with Butterpaper’s Stack
The magic really came to life when CockroachDB was incorporated within Butterpaper’s architecture. The seamless integration between CockroachDB and other tools like Dagster, DBT, and Airbyte was facilitated in the following ways:
Scalable Data Pipelines: With CockroachDB's inherent ability to scale, the data pipelines orchestrated by Dagster could manage increasing data loads efficiently.
Data Transformation with DBT: DBT’s ability to handle transformations was amplified when coupled with CockroachDB's rapid read-write capabilities, speeding up the data modeling processes.
Real-time Data Integration with Airbyte: Airbyte’s ELT processes fed data into CockroachDB in near real-time, ensuring the database was always populated with the latest data from various sources.
Measurable Benefits:
Post-implementation of CockroachDB, Agriwatch reported:
A 50% improvement in query response times due to optimized data distribution.
Almost 99.999% uptime, even during peak times.
A significant reduction in manual interventions for database maintenance and replication tasks.
CockroachDB proved to be the resilient backbone Agriwatch needed to keep up with the dynamic world of agricultural commodities. Its distributed nature, combined with other robust features, reinforced Agriwatch's data infrastructure, making it robust and future-proof.
Airbyte: Seamless Data Integration
In the realm of data integration, there have been ongoing debates on the efficacy of the ELT (Extract, Load, Transform) model over the traditional ETL (Extract, Transform, Load) approach. Airbyte, a modern, open-source data integration platform, has championed the ELT model. For Agriwatch, this choice was transformative. Here's why:
The Evolution from ETL to ELT: A Brief Overview
Traditional data integration followed the ETL model, where data is:
Extracted from source systems.
Transformed into a usable format, often outside of the destination datastore.
Loaded into the data warehouse or database.
The major constraint here is that transformation occurs before loading, necessitating powerful and often expensive middleware to handle large datasets.
On the other hand, ELT flips this approach. Data is:
Extracted from source systems.
Loaded directly into the destination system.
Transformed within the destination itself.
With the rise of powerful and scalable cloud data warehouses, this model allows for more flexibility and scalability, capitalizing on the data warehouse's processing capabilities.
Source-Centric Integrations: Airbyte’s design is predominantly source-centric. It focuses on extracting raw data from various sources without losing granularity. This ensures that the entire spectrum of source data is available in the destination, paving the way for diverse and dynamic transformation processes.
Rich Connector Ecosystem: Airbyte boasts a plethora of pre-built connectors, both for popular data sources and more niche ones. These connectors are geared towards ensuring high fidelity data extraction, prioritizing data integrity and consistency.
Real-time Data Loading: One of the features that set Airbyte apart is its capability for real-time data synchronization. By leveraging incremental updates, Airbyte can maintain a near-real-time sync between source data and the destination platform, ensuring that the loaded data is always fresh.
Flexibility in Transformation: Once data is loaded into the destination, users have the complete freedom to decide how and when to transform it. Given that Agriwatch dealt with diverse datasets, from weather patterns to commodity pricing, this allowed for tailored transformation logic for each dataset type. Moreover, as transformation logic could be modified without affecting the raw loaded data, it added a layer of resilience against errors and anomalies.
Scalability: ELT inherently benefits from the scalability of the data warehouse. By leaning into this model, Airbyte ensures that as Agriwatch’s data needs grow, the system can adapt without necessitating a comprehensive overhaul.
Airbyte vs Traditional ETL: Operational Benefits
For Agriwatch, Airbyte's ELT model provided multiple tangible benefits:
Performance: The load-first approach leveraged the advanced processing capabilities of modern data platforms like PostgreSQL, ensuring faster data transformations.
Flexibility: Since transformation took place post-loading, changes to transformation logic could be made without re-ingesting the entire dataset.
Cost-efficiency: By sidestepping the need for powerful middleware systems to handle transformations, there was a marked reduction in operational costs.
Data Integrity: With raw data loaded directly into the warehouse, there was minimal risk of data loss or dilution during the integration process.
Airbyte’s adoption of the ELT model, combined with its unique features, proved instrumental in modernizing Agriwatch's data integration. In a world where timely and accurate data can drive critical decisions, this modern approach ensured that Agriwatch was always equipped with the freshest insights, derived from data that was both comprehensive and trustworthy.
Conclusion: Harmonizing the Future of Agricultural Analytics
Navigating the technical intricacies of Agriwatch's transformation journey unveils an enlightening tale of how the right technological integrations can dramatically reshape the operational landscape of an enterprise. Agriwatch's metamorphosis, steered by the adept hands of Butterpaper Inc., reinforces the narrative that contemporary data engineering tools, when wielded adeptly, can orchestrate not just data, but success stories.
Benefits Realized:
Exponential Efficiency: With the adoption of modern technologies like Dagster, DBT, PostgreSQL, Airbyte, and the concept of Data Orchestration, Agriwatch experienced a surge in data processing speeds, driving timely insights and better decision-making.
Cost-Efficiency: Through streamlined operations and reduced redundancy, operational costs were drastically lowered, allocating resources more effectively.
Scalability: The modular nature of the solutions implemented ensured that Agriwatch can now adapt and grow without the hurdles they once encountered, setting them up for future expansions.
Informed Decision Making: By harnessing accurate, timely, and integrated data, Agriwatch now possesses the ability to make decisions rooted in comprehensive insights, propelling them ahead of competitors.
Customer Satisfaction: With faster report generation and in-depth analysis, Agriwatch can now offer unparalleled value to its clientele, fostering trust and solidifying its reputation in the market.
Looking forward, it is evident that the future of agricultural analytics is bright and boundless. As Agriwatch's transformation showcases, with the right partners and tools, any company can pivot from challenge-ridden operations to streamlined success. Here's to more organizations realizing the latent potential in their data and taking the leap towards an orchestrated future!
Comments